Insight led care: Using routine data for clinical prioritisation
There is growing backlog of NHS out-patient care and how to best manage risk in those waiting is a challenge. The diabetes team at Guy’s and St Thomas Hospital in collaboration with analytics team (Factor 50) defined and coded clinical rules of modifiable high risk that had emerged since a persons last clinic visit. This approach allows clinicians to objectively and pre-emptively identify and intervene in people who may be suffering harm whilst awaiting appointments. We demonstrated reduction in harm in high risk people and by also identifying 'lower' risk people facilitated patient initiated follow up to increase clinical capacity.
What was the problem?
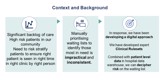
Covid-19 pandemic and many subsequent challenges to the NHS necessitated the block cancellation of massive volumes of outpatient activity , resulting in large outpatient follow backlog across the NHS.
Appropriate management of clinical risk in those waiting or who may be clinically deteriorating, awareness of the changing nature of patients conditions and appropriate use of clinical capacity are all key priorities clinicians. Identifying and prioritising patients on long outpatient waiting lists remains key challenge, with many of the existing methods for doing so being both impractical and inconsistent.
What did you do?
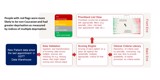
We developed, defined, coded and validated clinical rule sets of modifiable new onset risk since the patients last visit to objectively identify patients who may be suffering harm whilst on waiting lists.
Using this system we expedited appointments for >120 high risk patients and prevented harm in ~40% of this cohort. We reduced health inequalities as high risk people are disproportionately from ethnic minorities and areas of high deprivation
What did you learn?
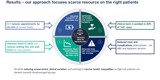
The Insight Led Care approach has had the unintended consequence of revealing opportunities to standardise assessments of hidden clinical risk and intervene pre-emptively to modify previously 'hidden' risk.
Our methodology is changing clinical practice and culture and can also help address health inequity as many people at highest risk are from ethnic minorities and or living in areas of high deprivation.
We can also use this method to increase clinical capacity (within existing resources) to see those people at highest risk, by reducing the frequency and type of clinical review/appointments and increasing patient initiated follow up for people with stable data.
What was the impact and what did you learn?
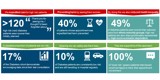
Prevented harm in ~40% of high-risk patients that were seen sooner than originally planned. Helped identify and reduce health inequalities. Reduce frequency of clinical review/appointments for people with stable data. Using this method for clinic cancellations and waiting lists ensure those at highest risk are prioritised.
How can you make sure it’s sustainable and what’s next?
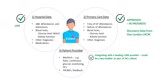
We have made a successful application to South East London Data Usage Committee to augment the dataset with Primary Care Data to improve the breadth and quality of risk scoring within the diabetes cohort. We are working on incorporating wearable glucose data to our clinical rules set/code to aid and augment prioritisation process.
References
Karalliedde J et al. A pragmatic digital health informatics based approach for aiding clinical prioritisation and reducing backlog of care: A study in cohort of 4022 people with diabetes. Diabetes Res Clin Pract. 2023. DOI: 10.1016/j.diabres.2023.110834
https://www.hsj.co.uk/awards/hsj-digital-awards-2023-driving-change-through-data-and-analytics-award/7